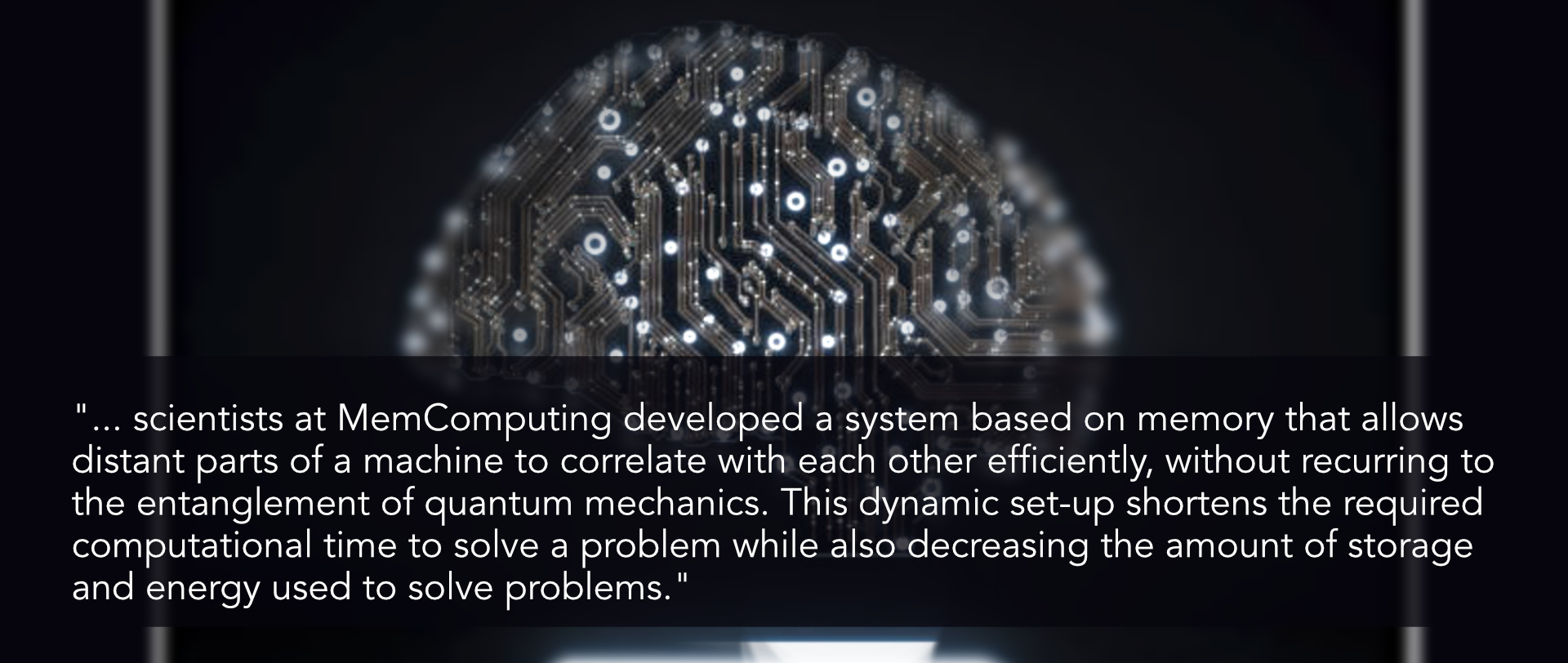
New computing systems
Today, we exploit known physical phenomena to efficiently compute difficult problems.
The most recent example is quantum superposition and entanglement used by quantum computers. In contrast to bits in today’s computers which can be either the so-called qubits in quantum computers can represent various possible combinations of at the same time. This admittedly pretty counterintuitive feature is called superposition and originates from the quantum theory. It basically allows quantum computers to calculate a vast number of potential solutions much quicker.The second phenomenon of quantum entanglement describes the fact that the state of one qubit influences the state of another one in a predictable way, even if they are separated by very long distances. Consequently, adding qubits increases the computing power of quantum machines exponentially whereas doubling bits in today’s computers means only a doubling of processing power.These physical phenomena combined are the basis of the massive computing power of quantum computers. However, to control these phenomena, the quantum computer has to be cooled down to cryogenic temperatures and any vibration must be avoided to not disturb the state of superposition and entanglement. For this reason, quantum computers won’t replace desktop computers any time soon.
Yet, it doesn’t mean that there aren’t other non-quantum physical properties that one may leverage to compute more efficiently. In fact, scientists at MemComputing developed a system based on memory that allows distant parts of a machine to correlate with each other efficiently, without recurring to the entanglement of quantum mechanics. This dynamic set-up shortens the required computational time to solve a problem while also decreasing the amount of storage and energy used to solve problems.
Others focus on developing memory modules based on a new architecture to reduce the performance gap between CPUs and RAM. Besides providing greater memory speeds, the memory developed by BlueShift Memory is also useful for AI applications based on deep learning, thereby leading the trend away from general purpose computers to task-optimized, specialized ones.